The traditional drug discovery process is infamously long, expensive, and unpredictable. On average, it takes 10 to 15 years and over a billion dollars to bring a single new drug from concept to market. Add to this a dismal success rate—with most candidates failing in clinical trials—and it’s clear why the pharmaceutical industry is hungry for innovation. Enter deep learning, a subset of artificial intelligence (AI) that is changing the game at every level of the drug development pipeline.
By leveraging powerful algorithms and massive biological datasets, deep learning is speeding up research, improving accuracy, cutting costs, and unlocking entirely new therapeutic avenues that were previously out of reach. This transformation is not theoretical—it’s happening now, and it’s poised to shape the future of medicine.
Early Discovery: Slashing Time and Costs
Deep learning’s most immediate impact is felt in the early phases of drug discovery. These stages—target identification, compound screening, and hit discovery—are typically slow and expensive. Traditional high-throughput screening requires testing thousands of compounds in a lab, a process that can take years and millions in resources.
Today, AI-powered models can evaluate chemical libraries in silico within days. Neural networks trained on existing molecular data can predict a compound’s drug-likeness, toxicity, solubility, and binding affinity with impressive precision. This drastically narrows down candidates for physical testing.
Companies like Insilico Medicine, Atomwise, and BenevolentAI use deep learning models, including convolutional neural networks and GANs (generative adversarial networks), to streamline lead discovery. AI also enables scaffold hopping and molecular optimization, modifying known molecules to enhance efficacy or reduce side effects.
In practice, this means labs spend less time on guesswork and more on promising leads—expediting the move from “maybe” to “medically viable.”
Predicting Protein Structures and Drug Interactions
Protein folding has long been one of biology’s great puzzles. Understanding a protein’s 3D structure is critical for developing drugs that can interact with it effectively. In 2020, DeepMind’s AlphaFold achieved a milestone by predicting protein structures with near-experimental accuracy using deep learning techniques.
AlphaFold’s contribution is monumental. Its models have revealed the structures of over 200 million proteins, covering nearly every known gene in existence. These insights are freely available through the AlphaFold Protein Structure Database, democratizing access and accelerating drug design globally.
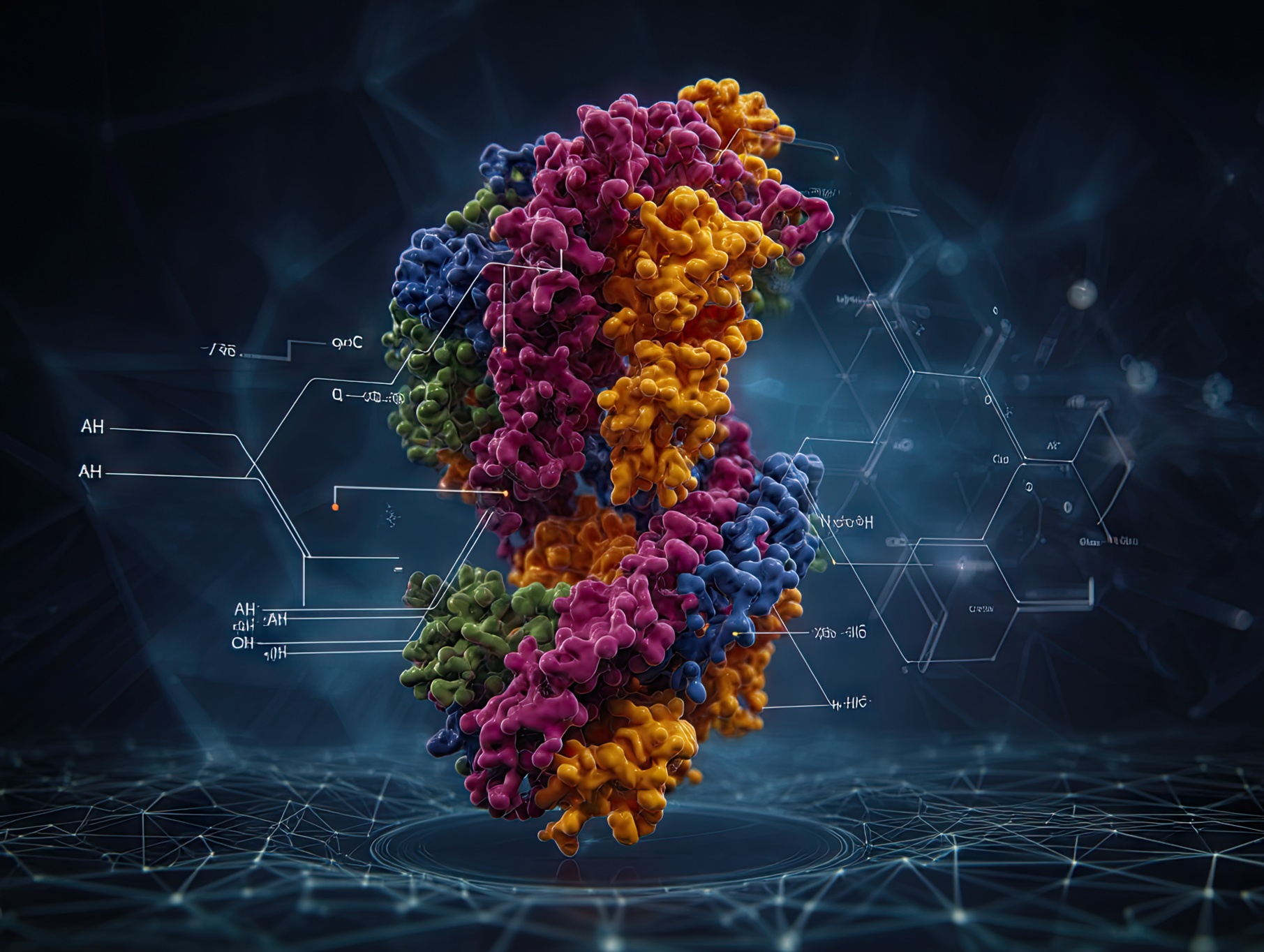
Beyond folding, deep learning models now simulate how candidate drugs interact with targets. Predictive tools assess absorption, distribution, metabolism, excretion, and toxicity (ADMET). This not only helps researchers design safer drugs but also reduces the likelihood of failure in late-stage development.
Companies like Exscientia and Cyclica are at the forefront, applying AI to develop bespoke molecules tailored to specific targets while minimizing off-target effects.
Transforming Clinical Trials and Drug Repurposing
Clinical trials are the most expensive and time-consuming phase of drug development. But deep learning is making them smarter and more efficient. AI helps identify ideal patient cohorts based on genetic and lifestyle data, enabling more targeted and personalized trials.
Adaptive trial designs powered by machine learning allow researchers to adjust parameters in real time based on incoming data. This not only increases success rates but also shortens timelines and improves ethical outcomes by reducing exposure to ineffective treatments.
AI also excels in drug repurposing, using existing drugs to treat new diseases. During the COVID-19 pandemic, deep learning models quickly analyzed known drugs for potential antiviral activity, helping to identify candidates like remdesivir and dexamethasone.
By uncovering hidden relationships within massive biochemical databases, AI is revealing opportunities that would take humans years to find, opening doors to faster, cost-effective therapies.
Merging AI with Experimental Biology
The integration of AI with experimental biology is creating a closed-loop innovation cycle. Algorithms generate hypotheses. Robotic systems test them. Results feed back into the models for further refinement. This iterative process accelerates discovery like never before.
Researchers are also combining multi-omics data—genomics, transcriptomics, proteomics, and metabolomics—to construct comprehensive disease models. Deep learning parses these complex datasets, identifying key biomarkers and therapeutic targets that single-data approaches might miss.
Such synergy between computational and experimental sciences is turning drug discovery into a dynamic, data-driven ecosystem.
Roadblocks and Considerations
Despite its promise, deep learning in drug discovery faces several challenges. One major issue is model transparency. Many deep learning models function as “black boxes,” making it difficult to interpret how they arrive at their conclusions—an issue that complicates regulatory approval.
Data quality and accessibility also remain obstacles. Training effective models requires vast, well-annotated datasets. Unfortunately, much pharmaceutical data is siloed, proprietary, or inconsistent. Open-access initiatives and public-private collaborations are working to improve data sharing and standardization.
Regulatory bodies such as the FDA and EMA are cautiously exploring how to validate AI-assisted drug development. Until there are clear guidelines and standards, adoption will proceed cautiously but steadily.
A New Era in Medicine
The use of deep learning in drug discovery is ushering in a paradigm shift. From accelerating early-stage screening to optimizing clinical trials, AI is rewriting the rules of pharmaceutical innovation.
This isn’t just about speed. It’s about precision, scalability, and accessibility. With deep learning, researchers can tackle rare diseases, personalize treatments, and reduce the financial burden of R&D. For patients, this means faster access to life-saving drugs. For the industry, it marks the beginning of a smarter, more sustainable future in healthcare.